Long Papers
(pending decision on uploading the full papers)
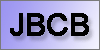 |
The papers will be published in the Journal of Bioinformatics and Computational Biology(JBCB). |
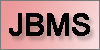 |
The papers will be published in the Journal of Biomedical Semantics(JBMS). |
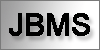 |
The extended papers will be published in the Journal of Biomedical Semantics(JBMS). |
Event Extraction with Complex Event Classification using Rich Features
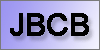
Pdf
Makoto Miwa, Rune S©¡tre, Jin-Dong Kim and Jun'ichi Tsujii
To capture biomedical phenomena more deeply, it is required to extract relations that are more complex than binary relations. To extract such complex relations, the BioNLP'09 shared task provided complex events; binding and regulation were provided as complex relations. To improve the biomedical event extraction systems, finding these complex events automatically is important; thus, we focus on the extraction of the complex events. In this paper, we propose an automatic event extraction system, which contains a model for complex events, by solving a classification problem with rich features. Our complex event detector performed better than the top system (in the shared task), and in overall performance, our system outperformed the top system.
Automatic Annotation by BioExcom for categorizing prior and new speculations in biological papers
Julien Descles, Motasem Alrahabi, Jean-Pierre Descles and Antoine Blais
Biological research papers are replete with speculative sentences. This paper presents the BioExcom software, an adaptation of EXCOM to the biology and biomedical fields, which annotates automatically all speculative sentences in full texts papers by the means of the Contextual Exploration processing. This annotation process is based on a fine semantic analysis of the multiple ways to express speculation in biology. Furthermore, BioExcom enables the automatically distinguishing of prior and new speculations in a biological paper. We argue that these annotations are useful for biologists' work, regardless of their domains of interest, helping them to evaluate quickly the content and new output of a paper. We discuss also some possible future applications of speculative sentences extraction and the CE processing in biology.
Analysis of syntactic and semantic features for fine-grained event-spatial understanding in outbreak news reports
Hutchatai Chanlekha and Nigel Collier
Previous studies have suggested that epidemiological reasoning needs a fine-grained modeling of events, especially their spatial and temporal attributes. While the temporal analysis of events has been intensively studied, far less attention has been paid to their spatial analysis. This article aims at filling the gap concerning automatic event-spatial attribute analysis in order to support health surveillance and epidemiological reasoning. In this work, we propose a methodology that provides a detailed analysis on each event reported in news articles to recover the most specific locations where it occurs. Various features for recognizing spatial attributes of the events were studied and incorporated into the models which were trained by several machine learning techniques. The best performance for spatial attribute recognition is very promising; 85.9% F-score (86.75% precision / 85.1% recall).
The application of an ontology design pattern for functional abnormalities to phenotype ontologies and the extraction of an ontology of anatomical functions
Robert Hoehndorf, Axel-Cyrille Ngonga Ngomo and Janet Kelso
Functions play an important role throughout biology. Although molecular functions are covered in the Gene Ontology, there is currently no publicly available ontology of anatomical functions. Ontological considerations on the nature of functional abnormalities and their representation in current phenotype ontologies show that we can automatically extract a skeleton for such an ontology of anatomical functions by using a combination of process, phenotype and anatomy ontologies. We provide an ontological analysis of the nature of functions and functional abnormalities. From this analysis, we derive an approach to the automatic extraction of anatomical functions from existing ontologies using a combination of natural language processing, graph-based analysis of the ontologies and formal inferences. Alternatively, we introduce a new relation to relate material objects to processes that realize the function of the object to avoid a needless duplication of processes already present in the Gene Ontology in a new ontology of anatomical functions. We discuss several limitations of the current ontologies that still need to be addressed to ensure a consistent and complete representation of anatomical functions and functional abnormalities.
The Value of an In-Domain Lexicon in Genomics QA
Pdf
Yutaka Sasaki, John McNaught and Sophia Ananiadou
This paper demonstrates that a large-scale lexicon tailored for the biology domain is effective in improving question analysis for genomics Question Answering (QA). We use the TREC Genomics Track data to evaluate the performance of different question analysis methods. It is hard to process textual information in biology, especially in molecular biology, due to a huge number of technical terms which rarely appear in general English documents and dictionaries. To support biological Text Mining, we have developed a domain-specific resource, the BioLexicon. Started in 2006 from scratch, this lexicon currently includes more than four million biomedical terms consisting of newly curated terms and terms collected from existing biomedical databases. While conventional genomics IR/QA systems provide query expansion based on thesauri and dictionaries, it is not clear to what extent a biology-oriented lexical resource is effective for question pre-processing for genomics QA. Experiments on the genomics QA data set show that question analysis using the BioLexicon performs slightly better than that using n-grams and the UMLS Specialist Lexicon.
Automatic Extraction of the Usage Information from the Component Words in Gene Ontology Terms to Enhance Consistency and Predictability
Seung-Cheol Baek and Jong C. Park
The Gene Ontology (GO) is a controlled vocabulary that has gone through constant changes, motivated primarily by the need to reflect the dynamic nature of knowledge it addresses and the need for usability improvement. A good policy on such changes would be to maintain consistency across terms and structures so as to highlight the missing parts that are likely to be added afterwards, or the unchanged parts to which a policy on usability improvement might not have yet applied. In particular, we argue that the component words inside terms must be used consistently across terms, in order to enhance the predictability of such terms, thus their usability as well. For this purpose, we propose a representation for word usage and a method for extracting it from GO and show its utility in identifying the direction of future changes readily as well as in enhancing the consistency of terms.
The CALBC Silver Standard Corpus - Harmonizing multiple semantic annotations in a large biomedical corpus
Pdf
Dietrich Rebholz-Schuhmann, Antonio Jose Jimeno Yepes, Erik M. van Mulligen, Ning Kang, Jan Kors, David Milward, Peter Corbett and Udo Hahn
The CALBC initiative aims to provide a large-scale biomedical text corpus that contains semantic annotations for tagged named entities of different kinds. The generation of this corpus requires that the annotations from different automatic annotation systems are harmonized.
In the first phase, the annotation systems from 5 participants (EMBL-EBI, EMC Rotterdam, NLM, JULIE Lab Jena, and Linguamatics) were gathered. All annotations were delivered in a common annotation format that included concept ids in the boundary assignments and that enabled comparison and alignment of the results.
During the harmonization phase, the produced results from different systems have been integrated into a single harmonised corpus ("silver standard" corpus) by applying a voting scheme. We give an overview of the processed data and the principles of harmonization - formal boundary reconciliation and semantic matching of named entities. Finally all submissions of the participants have been evaluated against the silver standard corpus. We found that species and disease annotations are better standardised amongst the partners than the annotations of genes and proteins.
The raw corpus is now available for additional named entity annotations. Part of the annotated corpus will be made available later for a public challenge. We expect that we can improve corpus building activities both in terms of the numbers of named entity classes being covered, as well as the size of the corpus in terms of annotated documents.
De-identifying Swedish Clinical Text -Refinement of a Gold Standard and Experiments with Conditional Random Fields
Hercules Dalianis and Sumithra Velupillai
In order to perform research on the information contained in Electronic Patient Records (EPRs), access to the data itself is needed. This is often very difficult due to confidentiality regulations. The data sets need to be fully de-identified before they can be distributed to researchers. De-identification is a difficult task where the definitions of annotation classes are not self-evident. We present work on the creation of two refined variants of a manually annotated Gold standard for de-identification, one created automatically, and one created through discussions among the annotators. These are used for the training and evaluation of an automatic system based on the Conditional Random Fields algorithm. Evaluating with four-fold cross-validation on sets of around 4-6 000 annotation instances, we obtained very promising results for both Gold Standards; F-score around 0.80 for a number of experiments, with higher results for certain annotation classes. Moreover, 49 false positives that were verified true positives were found by the system but missed by the annotators. Our intention is to make this Gold standard available for other research groups in the future. Despite being slightly more time consuming we believe the manual consensus gold standard is the most valuable for further research. We also propose a set of annotation classes to be used for similar de-identification tasks.
Enabling Recognition of Diseases in Biomedical Text with Machine Learning: Corpus and Benchmark
Robert Leaman, Christopher Miller and Graciela Gonzalez
Many lines of inquiry in biomedicine lead directly or indirectly to the prevention, diagnosis or treatment of disease. Utilizing text mining to further these lines of inquiry typically involves applying an extraction system including the recognition and identification (normalization) of the diseases mentioned as early steps in the pipeline. In recent years there has been a trend away from dictionary-based systems for the recognition of biomedical entities in favor of named entity systems based on machine learning for sequence tagging, such as conditional random fields. However, this trend has not yet extended to tagging diseases, despite a strong interest in disease entities, perhaps because of the difficulty in obtaining adequate corpora for training a machine learning system. We therefore introduce a new corpus (the Arizona Disease Corpus, or AZDC), derived from biomedical research abstracts, containing the necessary annotations for both named entity recognition and normalization of disease entities. We utilize this corpus to explore the performance of machine-learning based systems and dictionary match. We anticipate that this resource will prove valuable for mining disease-related knowledge from biomedical text, supporting the ability to translate our ever-increasing biomedical understanding into clinical applications and improved quality of life. The Arizona Disease Corpus (AZDC) can be freely downloaded*.
*http://diego.asu.edu/downloads/AZDC
Short Papers
Biological Event Recognition with Textual Induction
Yutaka Sasaki, Paul Thompson, John McNaught and Sophia Ananiadou
Pdf
This paper describes a supervised approach to the recognition of biological events, which combines statistical sequential labeling and symbolic event extraction rules. Bottom-up textual induction has been applied to generating event extraction rules. As an evaluation data set, we use a corpus of biomedical abstracts, in which biological events concerning gene regulation in E. coli and H. Sapiens have been annotated by a group of biologists. The event instance extraction performance has been evaluated using 10-fold cross validation. The experimental results show that named entity recognition (NER) and semantic role labeling (SRL) performance are close to annotator performance, as indicated by the inter-annotator agreement (IAA) scores, whereas automatic event extraction performance is around 28%, as compared to 40% IAA for exact manual event extraction.
A Re-evaluation of Biomedical Named Entity - Term Relations
Tomoko Ohta, Sampo Pyysalo, Jin-Dong Kim and Jun'ichi Tsujii
Pdf
Recent developments in biomedical text mining include advances at the reliability of named entity recognition as well as movement toward richer representations of the associations of named entities. We argue that this shift in representation should be accompanied by the adoption of a a more detailed model of the relations holding between named entities and other relevant domain terms. As a step toward this goal, we study named entity - term relations with the aim of defining a detailed, broadly applicable set of relation types based on accepted domain standard concepts for use in corpus annotation and domain information extraction approaches.
Demystifying protein annotations: toward increasing the compatibility of different corpora
Yue Wang, Jin-Dong Kim, Rune S©¡tre, Sampo Pyysalo, Tomoko Ohta and Jun'ichi Tsujii
Pdf
While there are a number of corpora with protein annotations, the annotations in different corpora are not compatible with each other. It is, however, not yet well understood how they are different and how the incompatibilities can be overcome. The situation discourages utilization of the corpora in a united way. It also indicates that even within individual corpora, the actual annotations are not well understood. We first compare the protein annotations of two corpora, GENIA and GENETAG. Based on the result, we propose several strategies to increase the cross-corpus compatibility. Experimental results show that the proposed strategies are effective and the incompatibility of the protein annotations between the two corpora can be removed if we properly consider their differences.
Sentence Simplification Aids Protein-Protein Interaction Extraction
Siddhartha Jonnalagadda and Graciela Gonzalez
Pdf
Accurate systems for extracting Protein-Protein Interactions (PPIs) automatically from biomedical articles can help accelerate biomedical research. Biomedical Informatics researchers are collaborating to provide meta-services and advance the state-of-art in PPI extraction. One problem often neglected by current Natural Language Processing systems is the characteristic complexity of the sentences in biomedical literature. In this paper, we report on the impact that automatic simplification of sentences has on the performance of a state-of-art PPI extraction system, showing a substantial improvement in recall (8%) when the sentence simplification method is applied, without significant impact to precision.
Effective Mining of Protein Interactions
Fabio Rinaldi, Gerold Schneider, Kaarel Kaljurand, and Simon Clematide
Pdf
The detection of mentions of protein-protein interactions in the scientific literature has recently emerged as a core task in biomedical text mining. We present effective techniques for this task, which have been developed using the IntAct database as a gold standard, and have been evaluated in two text mining competitions.
Posters
A Thesaurus and an Application Ontology for the Juvenile Arthritis Domain
Ernesto Jim¢¥enez-Ruiz, Rafael Berlanga-Llavori, Victoria Nebot, Antonio Jimeno-Yepes and Dietrich Rebholz-Schuhmann
Pdf
This paper is intended to present our experiences in the creation of a light weight thesaurus for the Arthritis domain and the reuse of this terminological resource to create an ontology to classify patients suffering different subtypes of Rheumatoid Arthritis, which is part of the Health-e Child project.
Comparison of methods for topic template queries in the biomedical domain
Antonio Jimeno-Yepes, Rafael Berlanga-Llavori and Dietrich Rebholz-Schuhmann
Pdf
Topic template queries are focused on a facet of a structured user information need. Examples of these topic templates are: the role of gene G in disease D and the interaction of proteins P1 and P2. These templates allow for multiple instances and some commonalities might be found which might provide improved retrieval on unseen instance queries of a template.
In this paper, we have analyzed two possible solutions that integrate the analysis of existing results based on query reformulation and the boosting of documents based on text categorization.
We show that both approaches produce interesting results when enough example queries are provided and that the boosting of retrieved document based on text categorization has a better performance.
Inference for bio-IE: GENIA meets EKOSS
Jin-Dong Kim, Steven Kraines, Weisen Guo and Jun'ichi Tsujii
Pdf
Information extraction for molecular biology (bio-IE) aims to find useful pieces of bio-molecular knowledge (bio-knowledge, hereafter) from natural language expressions in the literature, and to store them in a structured form accessible by computers. One example is protein-protein interaction (PPI) extraction (Bunescu et al., 2004), which has long been a primary task of bio-IE. Usually, a PPI is expressed by a pair of proteins. For example, from the text, "Secretion of TNF was abolished by BHA ...," the following PPI can be extracted:
P1: (TNF, BHA)
Recently, as the need grows for semantically rich bio-knowledge - e.g. Gene Ontology annotation (GOA) (Camon et al., 2004), pathways (Bader et al., 2006) - the structure of bio-knowledge to be extracted is becoming more complex. BioNLP'09 Shared Task (BioNLP'09, hereafter) (Kim et al., 2009) addressed IE for bio-molecular events (bio-events). In the task, a bio-event is expressed by a predicate-argument structure, where the predicate specifies the type of event, and the argument expresses various aspects of the event, e.g. theme, cause. From the sample text above, the following events can be extracted according to BioNLP'09:
E1:(Localization, T:TNF)
E2:(Neg regulation, T:E1, C:BHA)
As the structure of the target bio-knowledge becomes complex, a more elaborate language is required to describe extracted knowledge pieces. An elaborate description language can encode a considerable amount of information, allowing useful computation over the knowledge descriptions, e.g. inferences. For example, initially, the relation between TNF and BHA is not explicit by E1 and E2, but if the description language defines the theme relation to be transitive, then the relation can be induced:
E3:(Neg regulation, T:TNF, C:BHA),
which holds the meaning, "BHA negatively regulates (a unspecified activity of) TNF". This paper reports our preliminery implementation to show that if we properly define the semantics of description language, we can find implicit knowledge descriptions which are implied by existing ones, through inferences over those semantics.
ONER: Tool for Organization Named Entity Recognition from Affiliation Strings in PubMed Abstracts
Siddhartha Jonnalagadda, Philip Topham and Graciela Gonzalez
Pdf
Automatically extracting organization names from the affiliation sentences of articles related to biomedicine is of great interest to the pharmaceutical marketing industry, health care funding agencies and public health officials. It will also be useful for other scientists in normalizing author names, automatically creating citations, indexing articles and identifying potential resources or collaborators. Today there are more than 18 million articles related to biomedical research indexed in PubMed, and information derived from them could be used effectively to save the great amount of time and resources spent by government agencies in understanding the scientific landscape, including key opinion leaders and centers of excellence. Our process for extracting organization names involves multi-layered rule matching with multiple dictionaries. The system achieves 99.6% f-measure in extracting organization names.
Bio-medical Term Extraction on Simple Rule Language
Takashi Sinnou, Koichi Takeuchi and Nigel Collier
Pdf
For disease surveillance system, bio-medical term extraction is a key technology for a surveillance system of epidemic disease news from the Web. In the previous work we applied statistical learning model to extract terms from the Web site. The previous approach is good at extracting terms with high precision rates; however it is weak at extracting new terms that do not exist in the training data. Since we usually have new disease names a new term extraction approach with high coverage for unknown or low-frequent terms is needed. Recently, Simple rule Language (SRL), a rule-based word extraction language, is freely available. The SRL also has an developing environment called SRL editor. Thus we are constructing rules of bio-medical terms on the several language (such as English, Japanese, Thai and Vietnam) for the multilingual disease surveillance system. In this manuscript we confirm how we construct rules to extract Japanese bio-medical terms from Japanese news articles.
Literature mining for protein acetylation
Youngrae Kim, Hodong Lee and Gwan-Su Yi
Pdf
This paper presents a method of text mining to extract information of acetylation. Acetylation is known to be involved in epigenetic pathways for cancer, stem cell, and neural disease. However, previous effort that gathers information about acetylation only relies on experimental data, excluding the epigenetic mechanisms reported in the literature. To compile the epigenetic effects on biological pathways, we developed a preliminary method to extract information of acetylation target and site information from the PubMed abstracts.